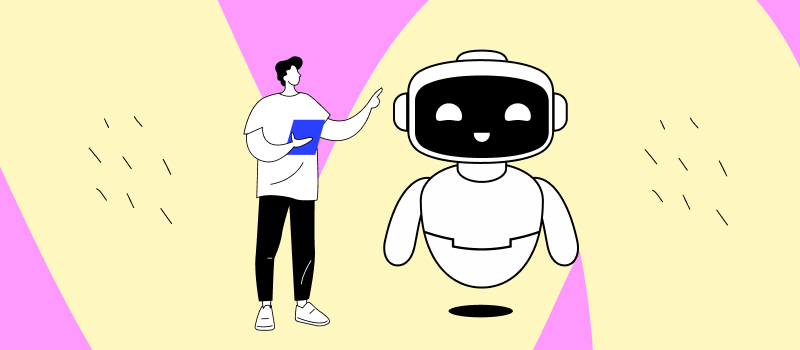
You couldn’t help but notice all this buzz around AI lately, uh? It seems like every week there’s a new article or podcast discussing how AI is going to revolutionize the way we lead teams and manage projects. For example, Gartner estimated that AI would perform up to 80 percent of the managers’ tasks by 2030. But, as with any shiny new technology, the conversation is far from one-sided.
We have the optimists who are all in on AI. They believe that machine learning and natural language processing can free up project managers to focus on more strategic tasks, like fostering team collaboration and driving innovation. Skeptics, in turn, raise some valid concerns about data quality and bias.
Who’s right? In this blog post, we’ll explore the transformative impact of AI on project management, examining both its benefits, challenges, and applications, and try to answer the most important question: “What does AI mean for the future of the profession?”
Summary
- Modern project management tools utilize GenAI, NLP, and machine learning.
- The integration of AI enhances decision-making by providing data-driven insights, enabling performance evaluations through real-time analytics, and facilitating training through personalized learning experiences. AI also aids budgeting by predicting costs and resource needs more accurately based on historical data.
- However, there are potential concerns associated with AI in project management, including the risk of over-reliance on technology and the need for human oversight to ensure that decisions align with organizational values and goals.
What Types of AI Can Be Used in Project Management and How?
Let’s stop thinking about AI as a magic wand to streamline your projects and take a look under the hood.
First up, we have Generative AI. It’s a subset of artificial intelligence that creates new content based on the data it has been trained on. Apart from processing data, Generative AI can produce text, images, music, and even code. It learns patterns and structures from vast datasets and uses this knowledge to generate new outputs that resemble the training data.
Here’s a simplified breakdown of how it works:
- Training. Generative AI models are trained on large datasets containing examples of the desired output. For instance, a text generation model might be trained on books, articles, and websites.
- Analyzing patterns. Next, the model analyzes the data to understand patterns, structures, and relationships within the content. It learns to predict what comes next in a sequence based on the context provided.
- Generation. Once trained, the model can generate new content by receiving prompts or inputs. It uses its learned knowledge to create coherent and contextually relevant outputs.
- Refinement. Many Generative AI models are also able to incorporate feedback and improve over time. As they receive more data and user feedback, their outputs become increasingly sophisticated.
Generative AI can help you create the basic structure for important documents like scope, schedule, and risk-mitigation plan, review the documents that are already created, and even offer risk management strategies that you will easily tweak to fit your project’s needs.
But remember that any AI is only as good as the data it works with. The very same Gartner that forecasted a bright future for AI found out that 85% of AI projects and models fail due to insufficient data quality.
If the underlying training data is flawed or biased, the generated content may reflect those issues. Moreover, Generative AI may not fully understand the specific context of a project, leading to outputs that may not align with the project’s goals or nuances.
Say, you decide to integrate a generative AI tool to generate weekly project status reports. The AI trained on previous project data and templates may fail to capture critical nuances regarding team dynamics and external project challenges, such as ongoing issues with vendors affecting timelines. It will result in a lack of proactive problem-solving and communication within the project teams, stakeholder confusion, and raised concerns about the managers’ capabilities.
Next on our list is Machine Learning (ML), which is supposed to help us make smarter decisions. Machine learning focuses on the development of algorithms and statistical models that enable computers to perform tasks without explicit programming.
How it works:
- Data collection. The first step in machine learning involves gathering relevant data. This data usually comes from databases, sensors, user interactions, or external APIs.
- Preparation. The collected data is pre-processed to clean it and make it suitable for analysis, which may involve handling missing values, normalizing data, and transforming variables.
- Choosing a model. Based on the problem to be solved, an appropriate machine learning algorithm is selected. Common types of ML algorithms include: supervised learning, trained on labeled data (input-output pairs) to make predictions, and unsupervised learning, which works with unlabeled data to find hidden patterns or groupings, and reinforcement learning, a model that learns by interacting with an environment and receiving feedback through rewards or penalties.
- Training the model. The chosen algorithm is trained using the prepared dataset. During this phase, the model learns to recognize patterns by adjusting its parameters based on the input data and the outcomes.
- Testing and validation. After training, the model is tested on a separate dataset to evaluate its performance.
- Deployment. Once validated, the model can be deployed into production for real-time predictions or decision-making.
- Monitoring and updating. If the model’s performance degrades or new data becomes available, the model may need retraining or fine-tuning.
As you can guess, ML algorithms would come in handy when you need to predict future outcomes, such as project completion times, budget overruns, or resource allocation needs, analyze feedback from team members or stakeholders (e.g., through surveys or communication channels) to gauge sentiment and morale, make better decisions based on trends and patterns rather than relying solely on intuition.
But not everything in the garden is rosy: despite being extremely costly, many machine learning algorithms, especially deep learning models, still operate as “black boxes,” making it difficult to interpret how decisions are made. Machine learning models can also inadvertently learn biases present in historical data, which leads to unfair or unethical outcomes.
Besides that, models trained on historical data may not adapt well to new conditions or unexpected changes in project scope, timelines, or resources.
Just imagine your company uses an AI tool to optimize resource allocation. The algorithm analyzes historical data, employee performance metrics, and workload to decide how to distribute team members, budget time, etc, across various ongoing and upcoming projects. Sounds logical, right?
However, the system trained on historical data that reflects past successes and failures is biased and may favour some teams or departments over others based on previous performance metrics.
If one department consistently got more resources because of past successes (even if those were due to external factors), the AI will also continue to allocate the same resources to that department, ignoring other teams that may have new ideas or potential.
Last but not least, let’s talk about Natural Language Processing (NLP). NLP is a branch of artificial intelligence that facilitates the interaction between computers and humans through natural language.
Natural Language Processing combines computational linguistics with machine learning, deep learning, and statistical methods to analyze and generate human language.
Here are some key components of the process:
- Tokenization: Breaking down text into smaller units, such as words or phrases.
- Part-of-speech tagging: Identifying the grammatical parts of speech for each word in a sentence (nouns, verbs, adjectives, etc.).
- Named entity recognition (NER): Identifying and classifying key entities within text, such as names of people, organizations, locations, dates, etc.
- Sentiment analysis: Determining the emotional tone behind a body of text, which can indicate positive, negative, or neutral sentiments.
- Text summarization: Condensing long pieces of text into shorter summaries while retaining essential information.
- Machine translation: Automatically translating text from one language to another.
These elements work together to help machines understand context, intent, and nuances in human language and save managers loads of time when it comes to comparing submissions, finding errors and omissions in project requirements, analyzing emails, chat messages, or feedback forms, and even replying to simple queries.
But there’s a wrinkle in that: human language is nuanced and context-dependent. NLP systems often struggle to understand context, sarcasm, idioms, or domain-specific jargon used within a project team.
For instance, in a software development project, developers might use slang or technical terms that are familiar to them but not easily understood by an NLP system. For instance, if a developer says, “We need to refactor this spaghetti code,” an NLP tool may misinterpret this as a literal request rather than understanding it as a call for code improvement.
The Promise and Reality
According to the Standish Group, only 35% of projects are considered successful, and the sheer amount of wasted resources and missed opportunities from the remaining 65% is staggering. So, it’s not surprising that thousands of CEOs and business owners jump on every opportunity to enhance the success rate of their projects and thus save trillions of dollars in value and benefits. The only question is whether AI is the solution they need.
In our research, we tried dozens of industry-leading project management tools, claiming they utilize AI, and found out that the applications of the advanced technology are limited, to say the least, and can be easily covered by the existing project management automation tools.
For example, AI promises to detect projects with a greater likelihood of success and provide a comprehensive overview of risks across the project portfolio, ensuring a balanced and unbiased approach to prioritization.
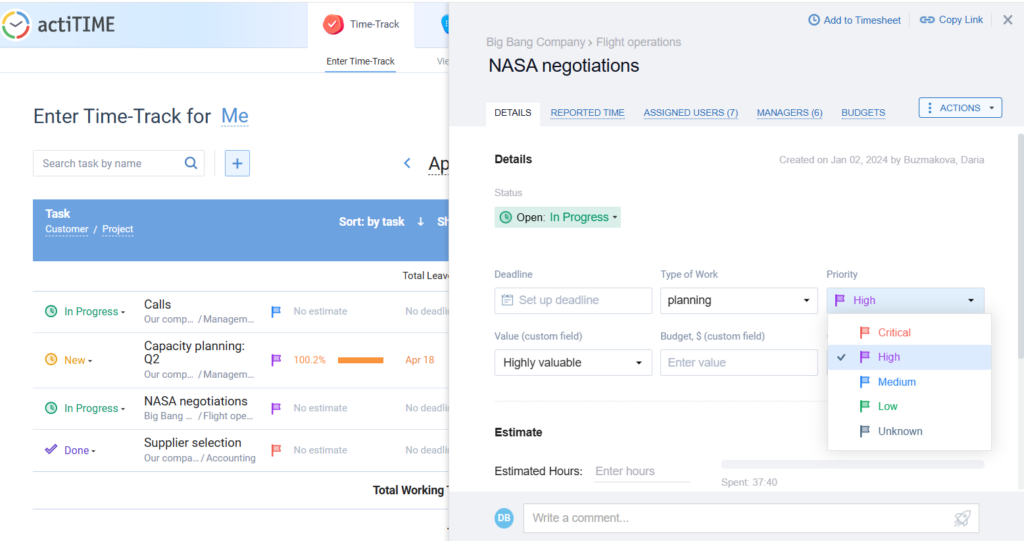
Setting task priority in actiTIME
In reality, the algorithms can’t boast anything beyond assigning most projects medium priority and leaving managers with the same task of estimating completion time and resources availability, as any task management tool would.
Then, AI proponents highlight the real-time tracking of project progress, anticipating potential issues, and automating responses to simple problems. And again, tools like actiTIME don’t require big data to warn you about an approaching deadline or potential budget overrun.
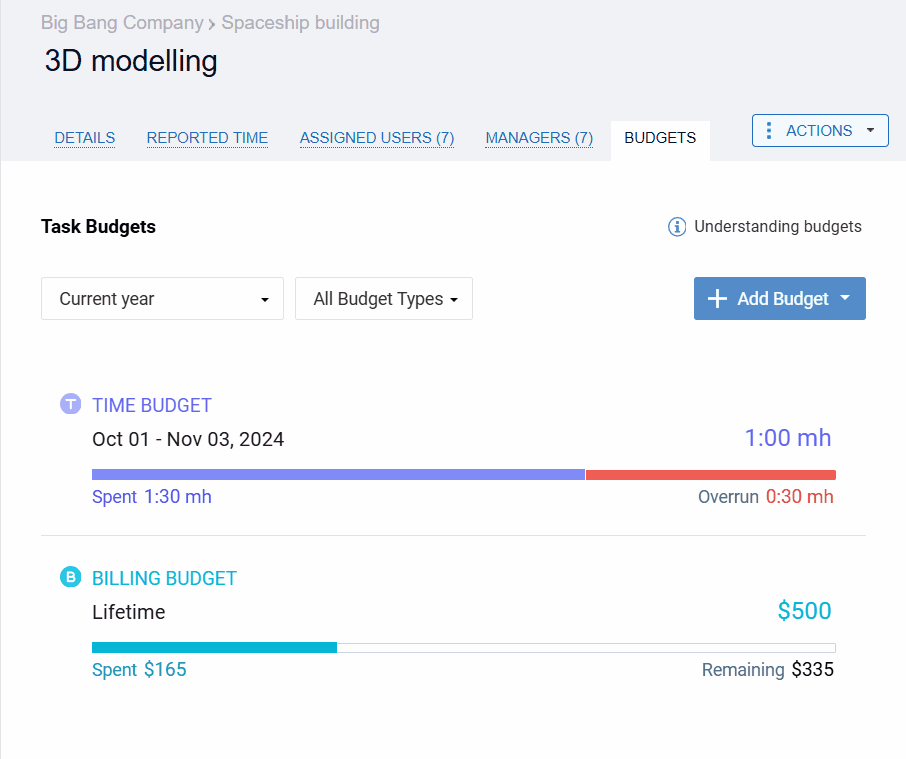
Budget overruns in actiTIME
What else? Advanced planning and reporting? These features are must-haves for the overall majority of project management tools in the market. And a study by Vlerick Business School and KU Leuven that simulated real-world budgeting problems found that AI lost to human participants in all the strategic finance planning challenges.
But is there anything AI can do for project managers right now, before the algorithms become truly sophisticated and the implementation gets really cheap? Sure!
Paul Bordeau from the AI IQ blog sees at least three areas where advanced algorithms can not just support, but replace the human workforce.
- Low-value tasks automation. Tools powered by Robotic Process Automation (RPA) can perform functions such as organizing meetings and generating project status reports. More advanced solutions also simplify note-taking, project ideas generation, and task summarization.
- Data structuring. The first step toward better decisions is creating a robust knowledge repository. This involves developing a comprehensive data strategy and capturing relevant data on various issues. AI excels at analyzing vast amounts of data quickly and accurately. So, it can identify patterns and provide insights that will help project managers make informed decisions.
- Team management. While some skeptics argue that AI cannot manage the human side of projects, recent surveys suggest otherwise. In fact, many employees would prefer to work for an AI-driven manager over their current human supervisors. NLP-powered tools can perform sentiment analysis to gauge the performance and feelings of team members. This analysis enables project managers to take proactive steps, such as providing additional communication or tailored feedback based on individual needs.
Embracing the Transformation
AI holds immense promise for transforming project management by enhancing selection, prioritization, planning, reporting, and communication. Moreover, the integration of Artificial Intelligence into management process is not just about adopting new tools; it represents a fundamental shift in how projects are executed and how the project managers’ role evolves.
The priorities shift from merely managing tasks and milestones to fostering high-performing teams and enhancing communication. So, the project manager of the future should transition beyond focusing solely on project deliverables, try to understand each team member’s strengths and weaknesses, and provide them with the resources they need to excel.